Insight into how technology will change roles and responsibilities
The situation
Finance and Risk functions across the banking industry are already over-stretched and find themselves under relentless pressure to service an ever-increasing portfolio of regulatory changes and demands whilst needing to devote more time to partnering with business users to ensure decision making such as optimal pricing, optimal capital allocation, optimal hedging, etc for driving maximum business value.
In this paper, we explore how we believe technology will change the role of the Finance and Risk professional, with a number of relevant examples and use cases.
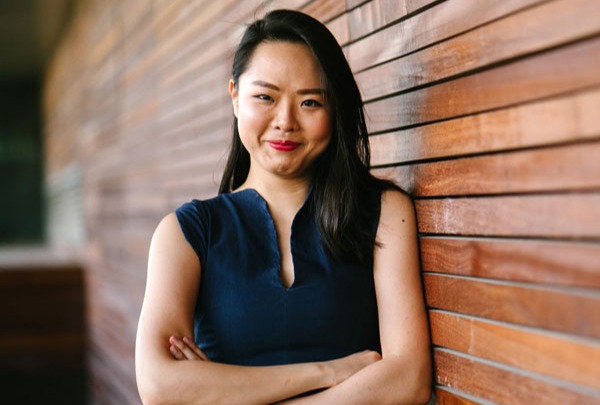
New technologies are resulting in the re-thinking of core processes and organisations doing things differently
Lack of investment in front to back systems, half-delivered implementations which are forced over the line by accepting more manual workarounds and a lack of desire to root-cause solve basic data issues mean the majority of the Finance and Risk functions time is spent physically generating the outputs rather than partnering with the business to maximise the value the outputs provide.
Existing daily activities need to be automated away to allow the focus on the value add tasks. Reconciliations, journal postings, sourcing data feeds, adjustments, creating graphs and decks of information, answering policy e-mails should be replaced by value add commentary, what-if scenario tools, forecasting models based on machine learning techniques, and managing the knowledge base of a chatbot.
A view of what the future role of the Risk Or Finance Professional will be, and the skills required to set the role up for success, is equally important and foundational in any transformation project. A transformation agenda needs to support this skill set through the delivery of the target Operating Model and target systems Architecture.
The good news is that you do not have to wait for the Transformation initiatives to deliver to make progress on the target skills required. The likely skills required can be introduced straight away as foundational and built on as the transformation initiatives layer in the technical and data capabilities.
Having this view also allows any future hiring or selection processes to be focused and informed on the skills needed in the future rather than entrenching the skills of today.
At NextWave Consulting we have leveraged our unique position of both leading-edge technology proponents combined with deep Risk and Finance experience to build a view of what these Future Skills are, why they are required and how they can be phase introduced across a number of pillars.
So what changes?
Case Study 1 : Report Production
Before: The monthly business performance report is due which requires e-mails to front office systems for extracts of financial and non-financial data. This data is then manually reviewed to make sure it covers the same time periods and whether there are any gaps or obvious deviations from prior months. In the meantime, the risk-weighted assets have undergone a material adjustment in the month-end process which means allocating the adjustment manually over the source data using a spreadsheet to ensure the report reconciles with the summary level information. The cost base information is received as a high-level aggregated number which requires another spreadsheet to allocate the costs down to individual business lines using historic averages. The report is then compiled into a presentation and sent off to the business via e-mail. The end-users raise questions on the data, dispute the values, and ask for a specific cut based on geography, which in turn requires repeating the whole process and gaining a new feed extract split by geography.
After: The team build an interactive dashboard in a visual designer and link this to a golden source of data. The golden source is automatically populated and reconciled so taken as complete and accurate, and at the lowest level of granularity to avoid aggregations and allocations. The business access the dashboard every day and are provided with an automatically natural-language-generated summary description of the changes since yesterday drawing their attention to the important moves. The business can filter and slice the data within the interactive tool whilst the conversation with the Risk team focuses on what other data to acquire to improve the business decisions and the models built by the risk team to forecast the future.
The Key Pillars Of Change
- Control & Oversight
- Professional Skills
- Change Leadership
- Problem Solving
- Data Science
- Business Expertise
- Communication Skills
- Leadership
Case Study 2 : Policy Advice
Before: The business email a group mail address with a question regarding the treatment of a product in a given country for risk purposes. The Risk team has a front line triage handler who goes through the email and directs it to the person best placed to answer if they do not have the answer to hand. This person may be out on vacation or not the correct person so the email gets forwarded around until an answer can be provided. There is little or no transparency on the cost or time to handle the response and little data to diagnose the underlying problem and fix at source.
After: The business enter their question into an automated natural language processing chat-bot. If the chat-bot has seen the question, or one similar, before and the confidence level is above a set threshold then a response is provided to the business straight away. If the answer is below the threshold then the question is routed to the most appropriate person based on the context of the question. The Risk individual then responds, or asks more questions, through the chat-bot which both answers the business questions and improves the chat-bot performance if the question is raised again. The chatbot also has a Risk nominated curator who randomly checks the chat-bot responses for accuracy and is given patterns of questions to drive pro-active fixes such as system fixes, training, or general information broadcasting.
Case Study 3: Top Level Capital Optimisation
Before: The CFO requires an optimal distribution of capital to maximise the return-on-equity across different businesses, countries, and products. This requires taking a starting position and asking each of the Risk business areas to perform their forecasting against this position, potentially sequentially to ensure bounding conditions such as liquidity ratios are not breached. Some areas are too complex to perform ad-hoc modelling so are reduced to simple models manually executed. Other areas have costs that scale with a potential investment which require further iterations to make sure the cost and revenue forecasts remain accurate. Any breach or challenge from the business requires repeating this process iteratively, at considerable manual effort cost and elapsed time, until an acceptable solution is found or time runs out.
After: Each of the Risk areas has generated models for forecasting which are based on a consistent data model, consistent data inputs and consistent factors. These models are all held in a centralised model repository that is capable of running the models for a given scenario without requiring manual intervention. The business define a scenario and the centralised orchestration tool performs a goal-seek calculation using all the forecasting models to identify the optimal solution and the sensitivities and risks of the solution.
Case Study 4: Monthly Forecasting
Before: Data requests are sent out at the mid-month point to get updates on the performance to date, any financial and non-financial indicators, and the sales pipeline. The sales pipeline data is discounted as largely irrelevant due to the sales teams only entering prospects they know are going to convert. The historic information is directly sourced from the front office systems so requires many hours of manipulation to map product types, business lines, and business geographies so they align with the submitted format. All of this is then processed through a spreadsheet that was inherited from the previous person that did the job and is not fully understood, but it gives a forecast for the remaining months of the year. Sometimes the spreadsheet throws out an anomaly that is not understood so a manual override is applied to bring it back into alignment.
After: Data feeds from the front office systems are received daily and populate the Risk shared datamart automatically overnight with all reference data automatically aligned. The Machine Learning suite automatically run against this new data to further train models, back-test for accuracy, and select whether any challenger models are given improved performance. The most accurate models for each line are automatically applied to produce the first draft forecast which is then reviewed with the business for the challenge. The sales pipeline data has been cleaned up and is now reliable as the business has learned the only way to influence the forecast is to improve the detail in the pipeline, and the accuracy of their sales prediction is now an objective fact. The forecasts are submitted with and without manual overlays to show the differences and build up confidence going forwards. In parallel, the Risk professional investigates alternate sources of data to further improve the forecasts, such as foot-fall statistics in the commercial retail sector, which increases the accuracy from 95% to 97% in that sector.
Interested in digital acceleration?
Subscribe to NextWave for occasional email updates on the latest technology, acceleration approaches, news and NextWave events. You can unsubscribe from these communications at any time.
Subscribe for our latest updates
Tags:
Digital & Automation
July 15, 2021